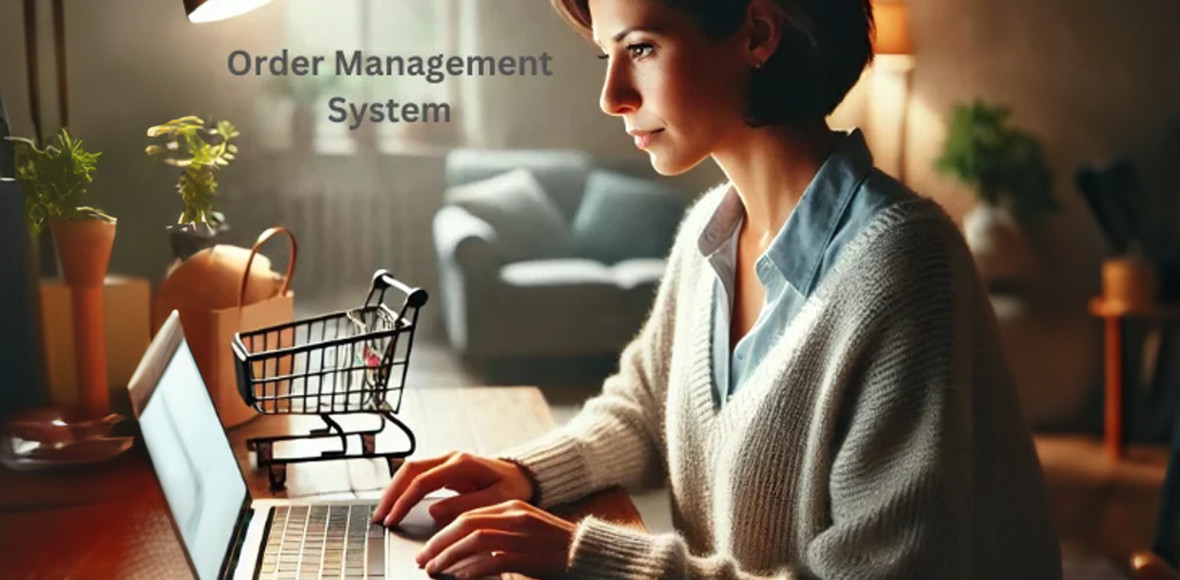
An Order Management System (OMS) serves as the foundation for e-commerce, retail, and logistics operations. It handles order processing, inventory management, shipping, and customer service. By adding predictive analytics to OMS, companies gain the power to guess future trends, make decisions, and adapt to market shifts.
Predictive analytics in OMS uses past data, statistics formulas, and machine learning methods to figure out what might happen next. By looking at old order patterns how customers act, and market trends, these prediction models can guess demand, spot supply chain problems before they happen, and suggest the best ways to run things.
Key Benefits of Predictive Analytics in OMS
1. Demand Forecasting and Inventory Optimisation
Predictive analytics has a major impact on OMS through its power to forecast demand. Old-school demand forecasting methods often depend on past sales figures, which might not grasp the ins and outs of today’s consumer habits. On the other hand, predictive analytics takes into account a broader set of factors such as seasonal changes, market shifts, marketing campaigns, and outside influences like the state of the economy or weather conditions.
Precise demand predictions help companies keep just the right amount of stock, cutting down on the chance of running out or having too much. This cuts storage expenses and makes sure customers get their orders, boosting their happiness and loyalty. Predictive tools can tell when to buy more stock, which items might see a jump in sales, and how to spread inventory across different places to work best.
2. Personalised Customer Experiences
Predictive analytics has a big impact on making customers happier by allowing for personalized interactions. When it looks at what customers have bought before how they browse, and what they like, predictive models can suggest products they might want, create special deals just for them, and guess what they’ll buy next. This personal touch does not boost sales but also makes customers feel closer to the business because their shopping feels like it’s made just for them.
Let’s say a customer often buys a certain brand of face cream every few months. The OMS can use predictive analytics to figure out when they might need more. This information can trigger emails or app alerts with special offers, making it more likely they’ll buy again.
3. Reducing Order Fulfilment Times
Predictive analytics has a big impact on cutting down order fulfilment times by making the whole process smoother, from when an order comes in to when it’s delivered. When forecasting models predict demand, they can put products in the best places across warehouses. This means items are stored closer to where people are likely to order them. This smart placement leads to shorter shipping distances faster deliveries, and lower transport costs.
What’s more, predictive analytics helps to spot holdups in getting orders out the door. By looking at data on how long it takes to process orders, how well warehouses pick items, and what causes shipping delays, companies can see where they need to make things better. They can then make changes to boost how well everything works overall.
4. Proactive Supply Chain Management
Supply chain problems can mess up a company’s ability to deliver orders when they’re supposed to. Using predictive analytics helps businesses see potential supply chain issues coming, like suppliers running late, shipping problems, or sudden shifts in what customers want. By using these prediction models, companies can spot weak points in their supply chain and take action to lower risks before they become big problems.
Let’s say a company uses predictive analytics to figure out how a supplier delay might affect their stock levels. The system might suggest other places to get supplies or changes to make in production plans. This way of staying ahead of issues lets businesses keep things running, cutting down on problems and making sure customers get their products without a hitch.
5. Dynamic Pricing and Promotion Optimisation
Predictive analytics enables businesses to use dynamic pricing strategies by looking at things like competitor pricing, customer demand, and market conditions. By grasping how these factors affect sales, companies can change prices on the fly to boost revenue and stay competitive. For example, predictive models can propose price hikes when demand is high or suggest discounts to increase sales during slow periods.
Also, predictive analytics can help make promotional campaigns better by finding which offers click with customers. By studying how past promotions perform, companies can create targeted campaigns that lead to more conversions and better returns on investment.
6. Enhanced Fraud Detection and Prevention
Fake orders and chargebacks create big problems for businesses. They hurt profits and make customers less trusting. Companies can use predictive analytics to get better at spotting fraud. This helps them find weird patterns in how people order things. For example, they might notice a sudden jump in expensive purchases or differences between billing and shipping info. By flagging orders that look fishy right away, businesses can check or cancel fake transactions. This protects their profits and keeps customers’ trust intact
7. Continuous Learning and Improvement
Predictive analytics has a powerful feature: it gets better with time. As companies gather and study more data, their forecasts become more precise. This lets businesses fine-tune how they predict and choose what to do. This ongoing learning helps firms stay quick on their feet. They can adapt to market shifts and what customers want with more accuracy.
What’s more, adding machine learning to predictive analytics means the models can change by themselves when new data comes in. They spot new trends or changes in how people buy things that might not be clear right away. This ability to change helps businesses stay ahead. They keep giving their customers what they need.
Implementing Predictive Analytics in OMS: Key Considerations
To make the most of predictive analytics in OMS, companies need to put money into the right tech, people, and ways to handle data. Here are some key things to think about:
- Data Quality and Integration: Make sure data from all key sources, like sales, inventory, and customer interactions, is clean, accurate, and combined into a main analytics platform.
- Scalable Technology: Pick an OMS that has built-in predictive analytics features or buy third-party analytics tools that work with your current systems.
- Skilled analysts and data scientists: Hire experts who can understand predictive analytics insights and turn them into real business plans.
- Security and Compliance: Protect sensitive customer and business data with strong security steps and follow data protection rules.
Conclusion
Predictive analytics in Order Management Systems is causing a revolution in how companies forecast, plan, and carry out their operations. It has an impact on businesses by offering deep insights into demand, inventory, customer behaviour, and supply chain dynamics. This allows companies to use data to make decisions that boost productivity, cut costs, and improve customer satisfaction. As the logistics and retail scenes keep changing, businesses that put money into predictive analytics will be ready to see change coming, act, and do well in the long term.